Understanding Compositional Structures in Art Historical Images using Pose and Gaze Priors
Written by Prathmesh Madhu
Contribution
Inspired from the pioneer work of Max Imdahl [1], our work focuses on generating image composition canvas (ICC) diagrams based on two central themes: (a) detection of action regions and action lines of an artwork (b) pose-based segmentation of foreground and background. In order to validate our approach qualitatively and quantitatively, we conduct a user study involving experts and non-experts. The outcome of the study highly correlates with our approach and also demonstrates its domain-agnostic capability.
Outcomes
- Proof of Concept for visual attention and similarity at how artworks are interpreted by humans and machines.
- Can help art historians towards their sophisticated art analysis. This helps in saving lot of time.
- A step towards understanding scenes without deep learning. This means not using annotated data; further using interpretable features for image retrieval.
- Ways to exploit existing pre-trained models and methods in computer vision for better interpretation of scenes
Limitations
- Currently, our approach works only for artworks comprising of protagonists (persons) in an image
- No benchmark data-sets available and no evaluation metric exists, so quantitative evaluation is very difficult.
- Our approach does not use state-of-the art gaze detection method, hence the gazes are not very precise.
- No working applications shown so far
Overview of the Methodology
Authors: Prathmesh Madhu*, Tilman Marquart*, Ronak Kosti, Peter Bell, Andreas Maier, Vincent Christlein (* represents equal contribution)
Preprint: Link coming soon
ACM Published Link : Accepted, VISART, 2020 (Link coming soon)
Code: https://github.com/image-compostion-canvas-group/image-compostion-canvas
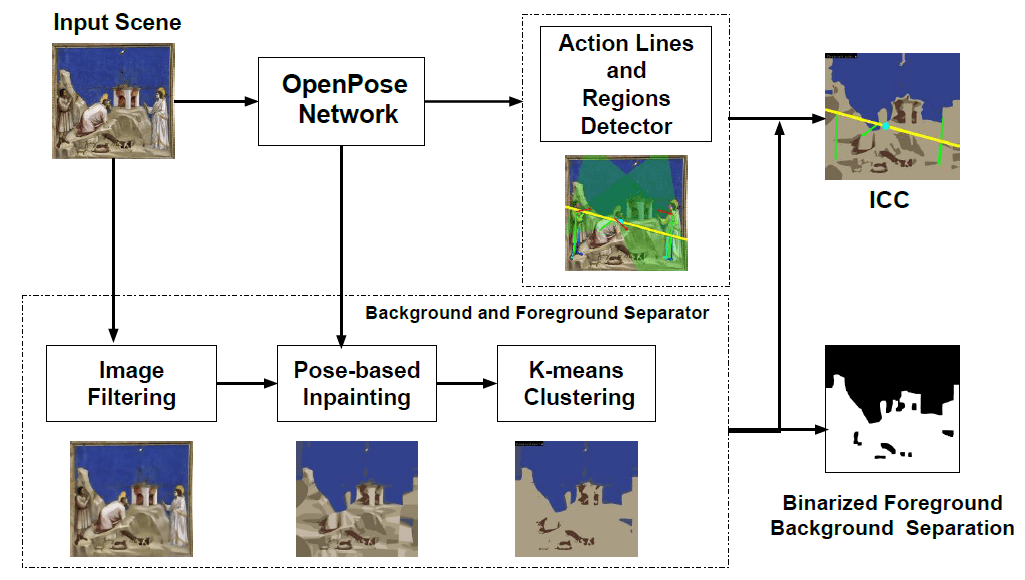
Definitions:
a) action lines : Global Action line (AL) is the line that passes through the main activity in the scene. This line normally is also aligned with the central protagonists. Local Action Line or Pose Line (PL) are the lines that represent the poses of the protagonists.
b) action regions : Action Regions (AR) is (are) the main region(s) of interest. More often than not it is the region where gazes of all the protagonists theoretically meet.
Our approach uses a pre-trained OpenPose [2] network, image processing techniques and a modified k-means clustering method. The pipeline of the proposed algorithm is shown in above figure.
Our method consists of two main branches: (1) a detector for action lines and action regions and (2) the foreground/background separator.
We use the estimated poses to detect pose triangles. We then propose a simple technique (gaze cones) to obtain gaze directions estimates without involving any training or fine-tuning. Combining this information, we draw a final ICC that estimates the image composition of the given scene under study.
Results
- Quantitatively, we proposed using HausDorff (HD) distance as an evaluation metric between lines and Euclidean distance between action regions.
- HD between all the annotators and ICC (ALL/ICC) is quite low (38 %) than the worst HD distance hence showing that the ALs of our ICC have very good correlation to all annotators.
- We observed that when the annotators’ agreement for the position of AR was higher / lower, our method predicted AR closer / farther to the labeled ones
- Qualitative comparison with User Study (Experts + Non-Experts)
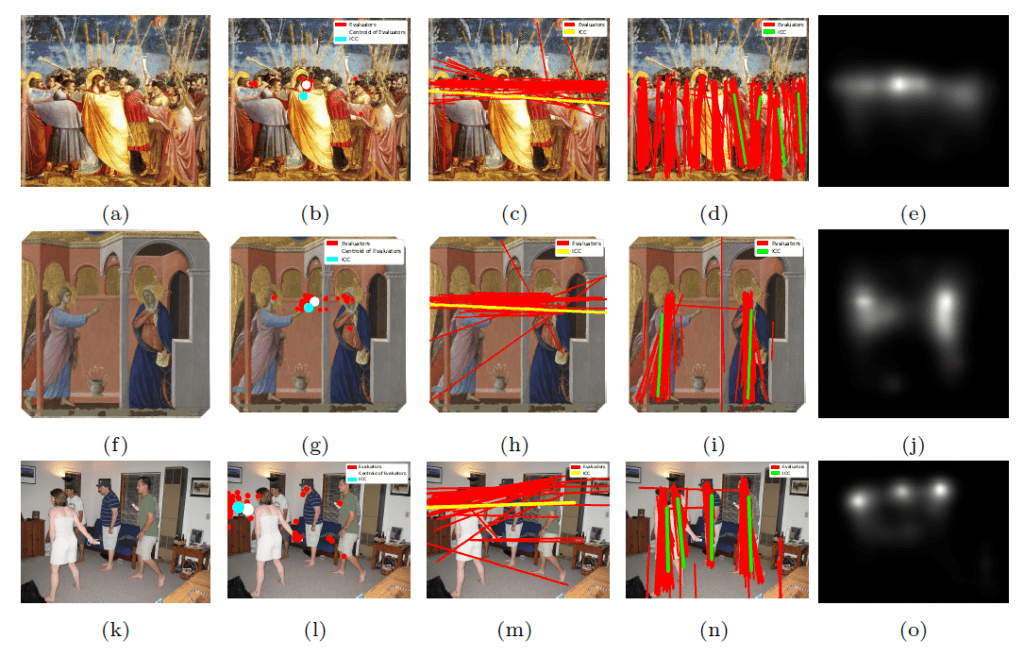
- Cross Domain Adaptability
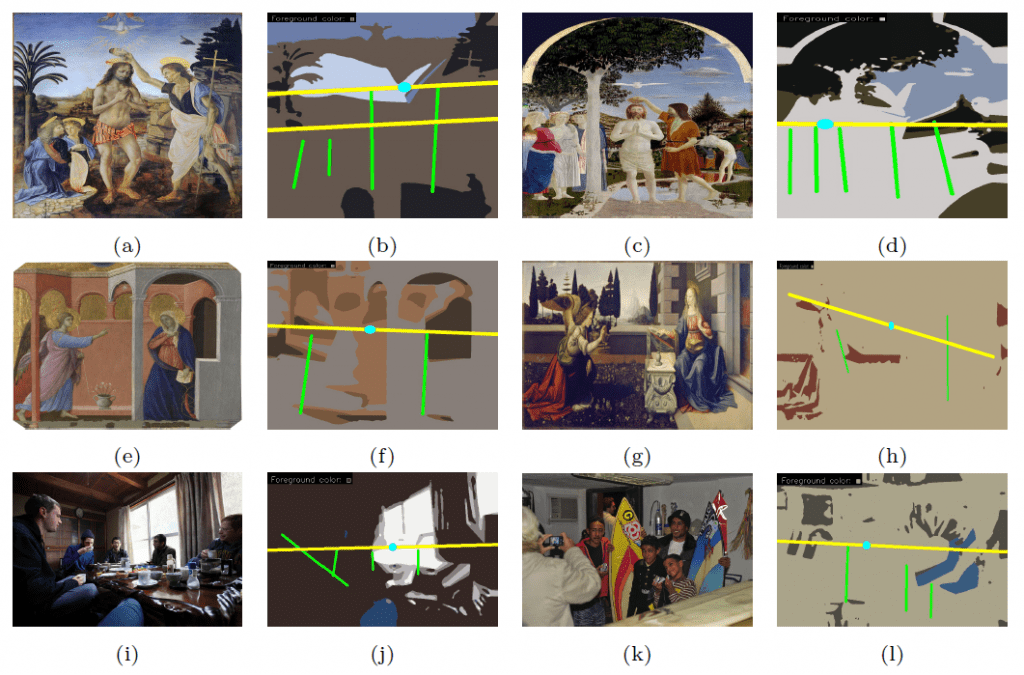
References:
[1] Imdahl, M.: Giotto, Arenafresken: Ikonographie-Ikonologie-Ikonik. Wilhelm Fink (1975)
[2] Cao, Z., Hidalgo, G., Simon, T., Wei, S.E., Sheikh, Y.: OpenPose: Realtime Multi-Person 2D Pose Estimation using Part A_nity Fields. arXiv:1812.08008 [cs] (May 2019)
[3] Lin, T.Y., Maire, M., Belongie, S., Bourdev, L., Girshick, R., Hays, J., Perona, P., Ramanan, D., Zitnick, C.L., Doll_ar, P.: Microsoft COCO: Common Objects in Context. arXiv:1405.0312 [cs] (Feb 2015)
Source: Pattern Recognition Lab, https://lme.tf.fau.de/news/understanding-compositional-structures-in-art-historical-images-using-pose-and-gaze-priors/